DataStories for House Price Predictions
Let's find us a house!
We are looking to buy a house in the beautiful Belgian city of Antwerp, the world's capital of diamonds and one of the top 10 fashion capitals of the world.
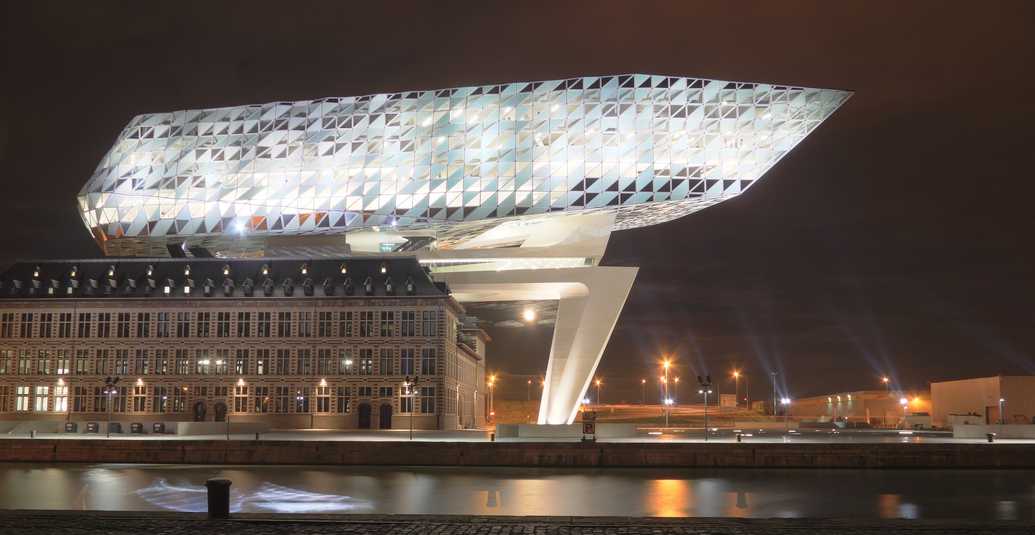
Luckily for us we've come across a large data set that contains many houses for sale in the Antwerp area. The data set contains all the information we would want to know such as the ground living area, how many cars can fit into the garage, how large the basement is or even what type of heating is installed.
Looking at the data set, it is quite complex and a bit messy. There's columns containing numerical values, text values and sometimes data is even missing.
You can spot the different attributes here in the data set:
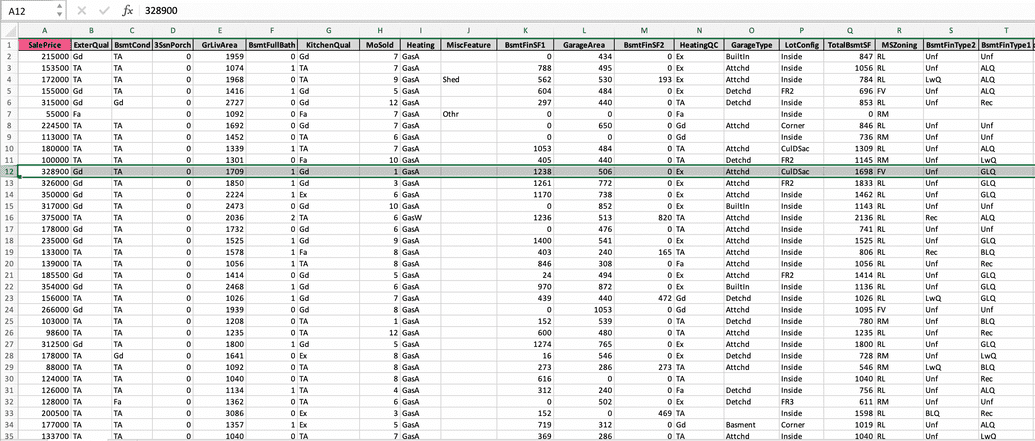
But for us, the most important metrics in this data set are the different prices for which these houses are put up for sale. We want to understand why a house costs as much or as little as it does. And if we can understand what drives the price, we can avoid buying a house that is extremely overpriced!
Let's create a Data Story
To start extracting insights out of this data set we'll start of with creating a new story in DataStories Platform. Generating a story can be done in 3 simple steps.
- We start by creating a new story and giving it a name and/or a description.
- We then upload the data set onto the platform and highlight our preferred KPI or Key Performance Indicator. This is the value we want to predict. In our case it will be the Sale Price of houses.
- We can change some extra story settings or leave everything as is and submit our story.
Normally it takes about 10 to 60 minutes, depending on your data set, for the platform to create a story. Once our story is ready, we can see a brief summary of our data. The dimensions of columns and rows used, date of story creation, the name of the author, etc...
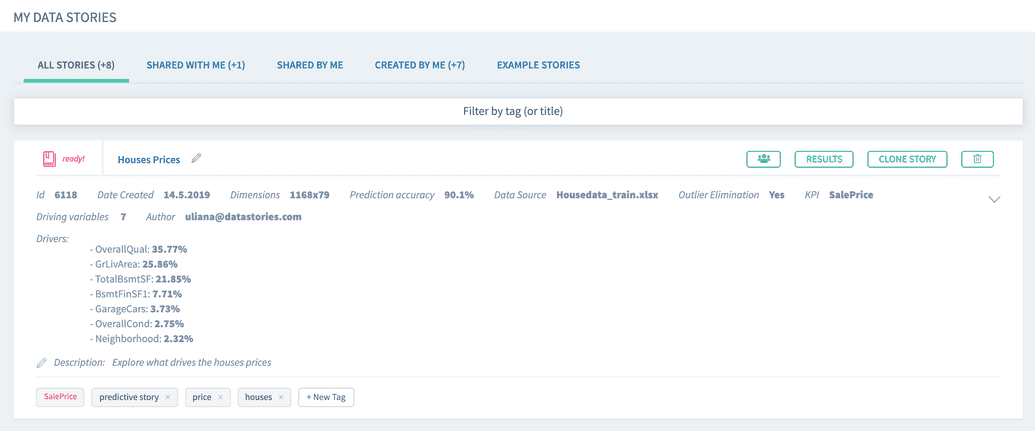
Clicking on results will open up our story. A Data Story consists of 12 chapters or as we like to call them 'Slides'. We'll go over most of them and see what we can learn.
Getting to know our Data
Before we start extracting insights from our data set it is important for us to understand how our data is structured.
When we upload a data set onto DataStories Platform we have already seen a brief summary of our data. The first couple of slides of our story, more specifically the Data Overview Slide, the Data Heatlh Slide and the KPI Slide will give you a deeper understanding of the data.
The Data Overview Slide will give you an overview about our data set. You can see general information such as the number of rows and columns that were present in our data set, the total amount cells, how much of the data was missing (e.g. empty cells).
On the Data Health Slide we dive deeper in the overall summary of our data. We already know that some data was missing or how many records were uploaded. But having lots of data is not enough, the quality is equally important and that is what we can analyze here.
The KPI Slide contains information about our target column SalePrice. Here we can see how our KPI, the Sale Price, appears in our data set.
For example we can see here that there are no missing values for our KPI, SalePrice, in the data set. This is good, it means the platform can use all the records in this data set to analyze what drives the sales price of a house!
Extracting Insights
Now that we have a clear understanding of our data we can start extracting valuable insights from it.
Moving up to the Sample Correlations Slide we can easily see certain connections between all the variables in our data set. We do this by connecting two columns with a line if the correlation among them is greater than a specified threshold.
We can see for instance that the Sale Price is strongly connected to certain variables: the Overall quality, External quality, Kitchen quality and Ground living area.
By highlighting the SalePrice you can explore the strongest KPI connection to the other variables. We can also highlight other variables and see which ones are strongly connected to them.
At Pair-Wise Plots we can observe in detail mutual information between the different variables and the Sale Price.
By analysing these graphs, you have a chance to find predictive behaviour for some variables and mark some areas of interest within your observations. It also might be that the plot won’t show any regular relations in the variables.
In this case we can see that if the overall quality increases, the price of a house also increases.
Predictive Insights
Now it's time to really dive deep into our story. The Predictive Models Slide generates some powerful insights on which variables are sufficient and necessary to predict the sale price of a house.
In our case, out of 79 variables, we can see that we only need 7 to accurately predict with an accuracy of 90.1%, the sale price of a house! The Overall Quality is picked up as the most important factor (35,8%) that has an impact on the final sale price, which seems logical.
At the What-Ifs Slide you can explore how the sale price of a house will change if we change the values of 1 or all of the 7 discovered variables that are the main drivers for the sale price.
One of the main features here is that you can quickly monitor the influence a certain driver has over the Sale Price. To truly interpret the impact of change of one driver, you should keep the others at the same values.
For instance, if we lower the Ground Living Area of a house, we can see that the price is intended to decrease, with the given configuration of other drivers:
You can also find out what the Sale Price would be should we give these drivers directly specified values. If we for instance know the values of these drives for a house that we are interested in, we can check whether the asking price is fair or not given that we have a predicted sale price at hand.
A reverse discovery would be: which characteristics (values) will a house have if I have a fixed budget to buy it.
Alternatively we can use the Maximize or Minimize KPI button's to quickly see what configurations of the discovered drivers should be to have a house selling for the highest or lowest price.
Conclusion
To summarize what we have learned and achieved with DataStories Platform:
- In total, our data contains 1 168 rows and 80 columns together with our KPI SalePrice, from which we selected 79 as inputs.
- 6.01% of our data was missing.
- We explored the data health of our data and it was rated as 'reasonable' according to the Data Health Slide
- We have examined all the variables and looked at their relations with the KPI - SalePrice.
- 7 features were found as the most important to predict the sale price of a house with 90.1% accuracy.
- We have discovered that Houses with higher 'Quality materials and Conditions' have a higher price.
- Houses that can accommodate two and three cars in the garage are in higher demand and are sold for a higher price.
- Houses that have greater 'Living area' and bigger 'Basement square feet' also show the trend of higher prices.
- The 'Neighborhood' has an impact on how expensive a house is.
These insights can be further formulated into actionable recommendations.
We learned what drives the sale price and we can make accurate assumptions on which sale prices are reasonable for the listed houses for sale in Antwerp.
Now it is up to us to get out there and start bidding. Welcome to Antwerp!